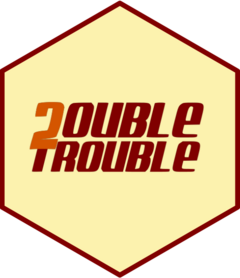
Find peaks in a Ks distribution with Gaussian Mixture Models
Source:R/ka_ks_analyses.R
find_ks_peaks.Rd
Find peaks in a Ks distribution with Gaussian Mixture Models
Arguments
- ks
A numeric vector of Ks values.
- npeaks
Numeric scalar indicating the number of peaks in the Ks distribution. If you don't know how many peaks there are, you can include a range of values, and the number of peaks that produces the lowest BIC (Bayesian Information Criterion) will be selected as the optimal. Default: 2.
- min_ks
Numeric scalar with the minimum Ks value. Removing very small Ks values is generally used to avoid the incorporation of allelic and/or splice variants and to prevent the fitting of a component to infinity. Default: 0.01.
- max_ks
Numeric scalar indicating the maximum Ks value. Removing very large Ks values is usually performed to account for Ks saturation. Default: 4.
- verbose
Logical indicating if messages should be printed on screen. Default: FALSE.
Value
A list with the following elements:
- mean
Numeric with the estimated means.
- sd
Numeric with the estimated standard deviations.
- lambda
Numeric with the estimated mixture weights.
- ks
Numeric vector of filtered Ks distribution based on arguments passed to min_ks and max_ks.
Examples
data(fungi_kaks)
scerevisiae_kaks <- fungi_kaks$saccharomyces_cerevisiae
ks <- scerevisiae_kaks$Ks
# Find 2 peaks in Ks distribution
peaks <- find_ks_peaks(ks, npeaks = 2)
# From 2 to 4 peaks, verbose = TRUE to show BIC values
peaks <- find_ks_peaks(ks, npeaks = c(2, 3, 4), verbose = TRUE)
#> Optimal number of peaks: 4
#> Bayesian Information Criterion (BIC):
#> E V
#> 2 -4833.369 -4312.906
#> 3 -4109.263 -3625.427
#> 4 -4124.051 -3397.111
#>
#> Top 3 models based on the BIC criterion:
#> V,4 V,3 E,3
#> -3397.111 -3625.427 -4109.263